Notebook / Appointments
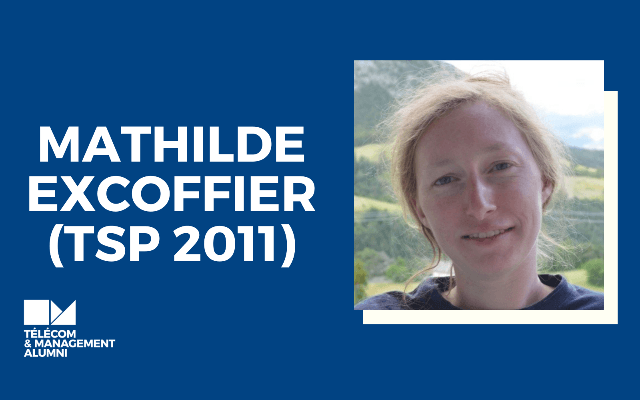
Portraits of Télécom SudParis - Portrait of Mathilde Excoffier (TSP 2011), Machine Learning Tech Lead at Facebook
Karolina Gorna (TSP, 2nd year student) meets Mathilde Excoffier (TSP 2011), Machine Learning Tech Lead at Facebook
1. Hello Mathilde, can you introduce yourself?
I graduated from Télécom SudParis, class of 2011, with a major in MSA (mathematics, statistics and applications). Between my second and third years, I did a "young engineer" year, during which I did an R&D internship at DCNS (now Naval Group) and another at CNRS in Japan. It was a special time, because Fukushima was taking place in Japan.
During this period, I developed a passion for applied mathematics, computer science, optimization and robotics. Following my engineering studies, I decided to do a thesis thanks to a grant from an organization between École Centrale Paris, École Polytechnique and Université Paris-Saclay. The subject of my research was "optimization under uncertainty". During this experience, I spent a few months in Montreal, at the invitation of a Canadian professor, to exchange ideas on our work. We were working on a similar theme. He was focusing his research on the application side, while I was more focused on the theoretical side, which enabled us to compare our work. Following my thesis, opportunities arose and I chose to move into industry. I worked in data science at Saint Gobain Recherche as a Research Engineer. Then I joined an international consulting firm where I was in charge of a Data Science team. I'm now starting a new adventure at Facebook, in Machine Learning.
2. Most of your career has been devoted to research. Why this choice, and what have you learned?
In preparatory school, many notions are explored in greater depth, but this is for the purpose of exercises. In engineering school, there's less in-depth study, leaving room for a wider range of things you can do, see or solve. When I embarked on research, I wanted to link these two aspects: to gain expertise in one field while being able to transpose it to other subjects. Solving problems for which you don't necessarily know the solution appealed to me. In prep school, we know there's a solution. In the world of research, on the other hand, we're moving towards the unknown, which is very stimulating!
3. You've traveled a lot for your work. Is there a different vision of science beyond our borders?
There are a number of differences. Firstly, the fields are perceived differently. Indeed, in France, the field of my thesis was categorized as computer science, whereas abroad, it was more mathematics. The French approach to mathematics is much more exclusive than in many other countries. I've been to Austria, Italy, England, Canada and Japan, and I've come to the same conclusion: every time, I've been working in mathematics laboratories.
Secondly, there is also the specificity of engineering schools, which is not always easy to explain internationally. However, the status of engineer is well recognized. In Canada, an association of engineers guarantees this credibility.
4. As a Lead Data Scientist, what does your day-to-day work involve?
My day-to-day work can be broken down into several different, complementary areas. First of all, there's the "business" aspect, which is very technical. Data Science is something relatively new (outside the academic world), very much in vogue and still little-known. The discipline is based on fundamental subjects such as mathematics, statistics and computer science. Then there's the "team management" part, a team mostly made up of engineers, whose aim is to help structure projects as well as guide people in their professional development. You have to be the team's voice within the company, to provide each member with the best possible support. Ultimately, it's important to make sure that everyone is more of an expert than I am! I would add, for the third axis, the "strategic development of skills for the company". Given the popularity of Data Science, this involves identifying high-potential projects, prioritizing subjects, and rallying support beyond the specialized team to ensure that projects are taken up by as many people as possible.
5. Are there any confusions between Artificial Intelligence, Machine Learning and Data Science?
It's a complicated question. I have a very technical profile and demand rigor and reliability. I think you have to take the time to see all the diversity that the data field has to offer, and not skip any steps.
I'd say that Machine Learning is the development of models that enable the machine to learn from a set of data in order to extrapolate information and deduce the future.
Data Science is the ability to make information speak from data. It's a vast field, from the development of statistical models and Machine Learning models to the visualization of analyzed results and the optimization of data management.
Finally, Artificial Intelligence is very broad for me, it's more of a concept, a goal towards which we're striving, that we're always pushing back further. I always compare it to the Turing test. Artificial Intelligence draws on the pre-cited fields, but also on so many others such as psychology, robotics, philosophy and morality.
I think the day when robots will dominate the world is not yet here, there's still plenty to do. What's more, statistics is a complex field, and by moving too fast, you can skew things very easily. What's more, I think we skipped the step of collecting our own data 20 years ago. Today, we finally have solid databases, from which we need to extract the information correctly.
5. Do you have any projects you particularly like or follow?
I follow all projects related to automatic language processing (ALP). Apart from artificial intelligence, there's already a lot to understand in linguistics. What's more, we're trying to integrate all this into models. The current trend in NLP is towards neural networks, which are not particularly easy to use. It's very interesting to note that there is a confrontation between language and models. Indeed, language is perceived as something very human, while models are seen as abstract elements. Sometimes, the way AI works enables us to better understand human behavior through the interpretation of language. In terms of specific projects, I can talk about disputes. In conflicts, disagreements don't often happen overnight; they take time. It's interesting to see the change in language and formulations that occurs in this process, and the neural networks can sense all this through an e-mail.
6. Taking stock, we've gone from your student days to the promising projects you're currently working on. Did you imagine that your current situation would be similar to what it was when you were a student?
No, I never imagined it. I've always had a certain professional ambition, wanted to do interesting things and be in a field where I could learn. In that sense, I'm happy where I am. In my case, the direction I've taken represents a good opportunity for someone who wants to keep their skills sharp at the technical level, while being close to high-level issues, in other words directly linked to business needs. What I learned during my studies has served me well, and I'm still learning. It's really satisfying!
7. Does your busy working life leave any room for passions?
This point is not necessarily obvious. Before the confinement, I travelled again to Canada. You could say that travel is a passion. What I enjoy most is fitting into a given place.
As for my other passions, I also enjoy mountain activities such as mountaineering and hiking, as well as the escape games I recently discovered.
8. There's that drive to solve problems and climb to the top! Do you have any advice for students wishing to follow in your footsteps?
My first piece of advice is not to neglect your courses in engineering school! For my part, I took what I wanted from the classes. However, I now realize that there was a real context to these courses and that the teachers were available to exchange ideas with them. I'm really glad I kept my handouts and the contact with the teachers.
My second piece of advice is to try. I'm not particularly fond of proverbs such as "where there's a will, there's a way". I don't think it's relevant. I'd rather say "when you want, you try". You have to be a little daring, it doesn't take much. Of course, sometimes things don't work out. In research, I've already contacted researchers with whom I wanted to collaborate, without getting any feedback. It doesn't matter, there were others who were interested. To sum up, when you have a desire, you have to try.
9. Any last words?
Right now, for students in particular, the word that comes up a lot is resilience. It's a term that's sometimes overused, but the idea of managing to build something despite the situation remains essential. For me, what's important in the concept is patience, the fact of trying. In my case, the events at Fukushima called into question my internship and all my work. There were a lot of twists and turns, and a lot of uncertainty. It can be discouraging. Right now, even if it's not the same thing, I'm aware that the situation is a bit frustrating. You have to try as hard as you can to stay active. For my part, I've tried to do what I love, and to do it well, and I've found the path I'm on now. One thing's for sure: there isn't just one path. That's why it's so important to explore the different possible fields open to us.
No comment
Log in to post comment. Log in.